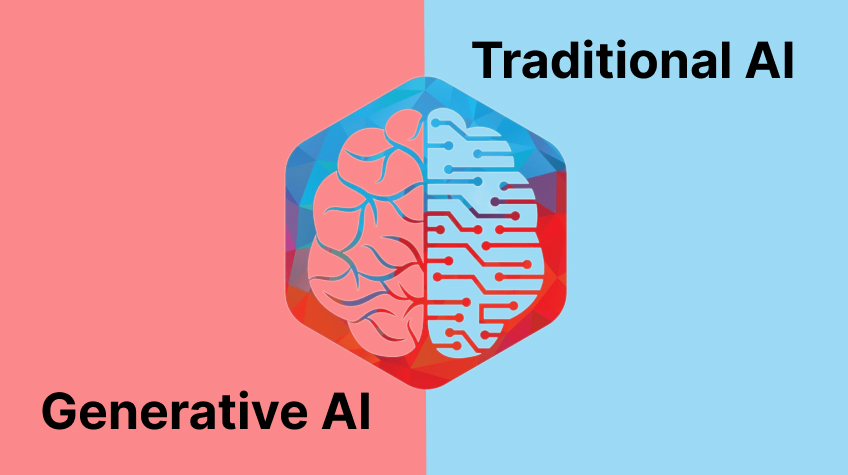
Generative AI vs Traditional AI ever-evolving realm of artificial intelligence, a profound shift has occurred that promises to redefine the boundaries of what machines can achieve. Traditional AI, with its rule-based systems and data-driven approaches, laid the foundation for many practical applications, from recommendation engines to predictive analytics. Yet, it remained confined to the rigidity of algorithms and structured data. Enter Generative AI, a game-changer that heralds a new era of machine capabilities.
It’s the creative powerhouse that challenges the status quo, enabling machines not just to analyze and interpret data but to generate entirely new content – be it art, music, or even human-like text. In this exploration of “Generative AI vs. Traditional AI,” we delve into the fundamental differences and the revolutionary potential that Generative AI brings to the table. Join us as we unravel the future of AI, where creativity meets computation and innovation knows no bounds.
Understanding Traditional AI
1. Definition and Characteristics
● Definition of Traditional AI:
○ Traditional AI refers to the early paradigms of artificial intelligence rooted in rule-based systems and data-driven algorithms.
○ It relies on predefined rules and structured data to make decisions and solve problems.
● Core Principles:
● Rule-Based Systems:
○ Traditional AI heavily relies on rule-based systems, where a set of explicit rules govern the behavior of the AI system.
○ These rules are typically crafted by human experts who have domain knowledge.
● Expert Systems:
○ Expert systems are a prominent example of Traditional AI. They emulate the decision-making abilities of human experts in a particular field.
○ Based on facts and heuristics, expert systems make inferences and provide solutions or recommendations.
● Limitations of Traditional AI in Handling Complex Tasks:
● Limited Adaptability:
○ Traditional AI systems struggle to adapt to new or unexpected situations. They are rigid and incapable of discovering from experience.
○ Any changes or updates to the system require manual adjustments to its rules.
● Dependency on Structured Data:
○ Traditional AI relies heavily on structured, well-organized data. It needs to work on messy data, which is expected in real life.
○ Handling unstructured data, such as natural language text or unformatted images, is a significant challenge.
● Scalability Issues:
○ As tasks become more complex, the rule-based approach becomes less effective. Managing a vast number of rules for intricate problems becomes impractical.
● Lack of Creativity:
○ Traditional AI lacks the ability to generate creative answers. It operates within the confines of rules and patterns that have been predetermined.
○ Innovative problem-solving and creative content generation are beyond its capabilities.
● Inability to Handle Uncertainty:
○ Traditional AI struggles with uncertainty and ambiguity. It often fails when faced with incomplete or contradictory information.
○ Expert systems may provide inaccurate recommendations if the underlying knowledge base needs to be completed or updated.
Traditional AI, characterized by rule-based and expert systems, has been foundational in AI development but is constrained by its rigidity, dependence on structured data, and limited adaptability, making it less suitable for handling complex and dynamic real-world tasks.
2. Data-Driven Approaches
● Reliance on Structured Data:
○ Traditional AI predominantly operates with structured data, which is highly organized and formatted.
○ It depends on well-defined variables, tables, and databases where data is categorized and labeled.
○ This structured format facilitates rule-based systems and algorithms, making processing information easier for Traditional AI.
● Supervised Learning:
○ Supervised learning is a common approach in Traditional AI where algorithms are trained using labeled data.
○ The AI system learns to make forecasts or decisions by associating input data with known outcomes.
● There are many applications for supervised learning, including:
○ Classification: Sorting data into predefined categories (e.g., email spam detection).
○ Regression: Predicting numerical values based on input data (e.g., house prices are predicted based on the size of a house and its location).
● Challenges with Data Quality and Quantity:
● Data Quality:
○ Traditional AI’s effectiveness is heavily contingent on data quality. Only correct or entire data can lead to precise results.
○ Ensuring data accuracy, consistency, and reliability can be resource-intensive and challenging.
● Data Quantity:
○ Traditional AI often requires a large volume of data for training, particularly in supervised learning.
○ Acquiring and preparing substantial datasets can be time-consuming and expensive.
● Data Bias:
○ Training data can contain biases that can affect AI models. Traditional AI can inadvertently perpetuate biases present in historical data.
○ Mitigating bias in AI systems is a crucial ethical matter.
● Data Maintenance:
○ As data evolves, Traditional AI systems may become less accurate or relevant. Periodic updates and upkeep are required to keep the models up-to-date.
● Scalability:
○ When dealing with large datasets, Traditional AI approaches may face scalability issues.
○ Processing and analyzing vast data can strain computational resources and extend processing times.
● Generalization:
○ Traditional AI models, particularly in supervised learning, may need help to generalize well to new, unseen data.
○ Overfitting (model memorizing the training data) or underfitting (model oversimplification) are everyday challenges.
Traditional AI’s reliance on structured data, use of supervised learning, and the challenges associated with data quality, quantity, bias, and maintenance shape its approach to solving problems. These challenges highlight the need for robust data management and ongoing model refinement in traditional AI applications.
The Emergence of Generative AI
1. What is Generative AI?
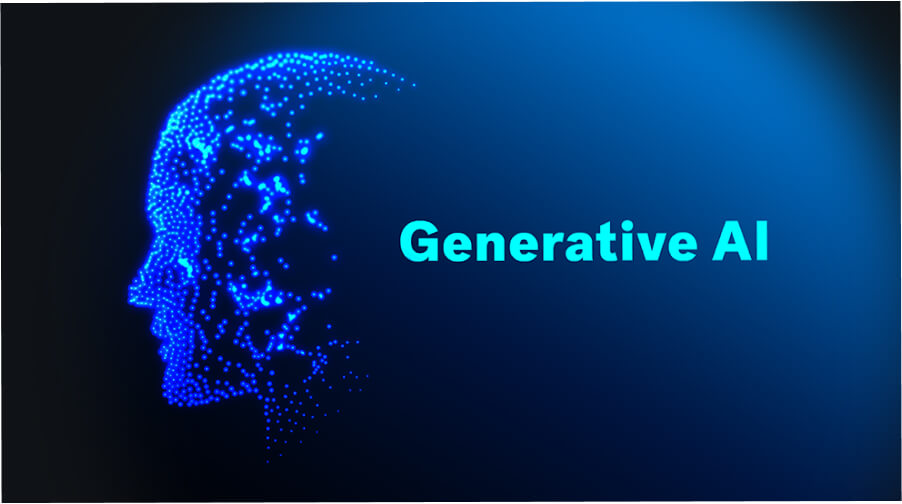
Generative AI represents a rebellious jump in artificial intelligence, enabling machines to analyze data and create entirely new content. At its core, Generative AI employs algorithms and models that can generate data, such as images, text, or even music, with a level of creativity and innovation previously thought to be exclusive to humans.
GANs (Generative Adversarial Networks) and VAEs (Variational Autoencoders) are two fundamental concepts in Generative AI. GANs pit two neural networks against each other, generating and evaluating content and fostering creative competition. VAEs, on the other hand, use probabilistic techniques to generate content while retaining the ability to manipulate data distributions, adding versatility to the creative process.
2. Creativity and Innovation
● Creative Capabilities of Generative AI:
○ Generative AI exhibits an exceptional capacity for creativity, enabling it to produce novel and imaginative content.
○ It can generate content across various domains, including art, music, and text, often surprising and inspiring with its output.
● Examples of Generative AI in Creativity:
● Art Generation:
○ Generative adversarial networks (GANs) have been used to create striking, original artwork. Artists and AI collaborate, with the AI generating images that artists then enhance or modify.
● “Edmond de Belamy,” a portrait generated by GANs, even fetched over $432,000 at auction.
● Music Composition:
○ Generative AI can compose music in various styles and genres. AI algorithms analyze existing compositions to generate entirely new pieces.
○ Composers and musicians are increasingly collaborating with AI to explore new musical landscapes.
● Text Generation:
○ AI-powered language models like GPT-3 can produce coherent and contextually relevant text, from news articles to creative storytelling.
○ AI-written content is being used in journalism, marketing, and even generating conversational chatbots.
● Fostering Innovation in Content Creation:
● Unleashing Unconventional Ideas:
○ Generative AI breaks creative boundaries, encouraging artists, writers, and musicians to experiment with unconventional ideas.
○ It inspires new forms of expression and challenges the status quo in different innovative enterprises.
● Efficiency and Personalization:
○ Generative AI streamlines content creation by automating repetitive tasks. This allows creators to focus on purifying and customizing the content they generate.
○ It accelerates content production while maintaining high quality.
● Cross-Domain Inspiration:
○ Generative AI can cross-pollinate ideas across different creative domains. For instance, an AI-generated artwork might inspire a musician to compose a matching soundtrack.
● Such cross-domain collaboration fuels innovation by merging diverse artistic elements.
Generative AI is a testament to the fusion of technology and creativity, reshaping traditional creative processes and fostering innovation across various content creation fields. It enables humans and machines to collaborate, pushing the limits of what’s artistically viable.
Generative AI Vs. Traditional AI: Use Cases and Applications
1. Traditional AI Use Cases
● Recommendation Systems:
○ Traditional AI is widely used in recommendation systems, suggesting products, content, or services established on consumer behavior.
○ Examples include Netflix’s movie and Amazon’s product recommendations, enhancing user experience and driving sales.
● Predictive Analytics:
○ Traditional AI excels in predictive analytics, leveraging historical data to make forecasts and informed decisions.
○ Businesses use it for demand forecasting, stock market predictions, and risk assessment in the finance and insurance industries.
● Fraud Detection:
○ Traditional AI detects fraud by analyzing transaction data and identifying fraud by analyzing transaction data and identifying anomalies.
○ Banks and credit card companies rely on AI algorithms to protect against fraudulent transactions.
● Natural Language Processing (NLP):
○ In NLP, Traditional AI is utilized for duties like sentiment analysis, chatbots, and language translation.
○ Virtual assistants like Siri and chatbots in customer support are examples of Traditional AI applications.
● Image Recognition:
○ Traditional AI can classify and identify objects in images, making it valuable in healthcare (diagnosis from medical images) and security (facial recognition).
● Manufacturing and Quality Control:
○ Traditional AI is used in manufacturing for quality control by inspecting products for defects or ensuring assembly line efficiency.
● Customer Relationship Management (CRM):
○ Traditional AI enhances CRM systems by analyzing customer data to personalize marketing strategies, leading to better customer engagement and loyalty.
● Supply Chain Management:
○ Traditional AI optimizes supply chain operations by forecasting the market, managing inventory, and enhancing logistics.
Traditional AI finds extensive applications across industries, excelling in tasks that involve structured data, rule-based systems, and well-defined problem domains. It enhances efficiency, decision-making, and user experience in various domains.
2. Generative AI Applications
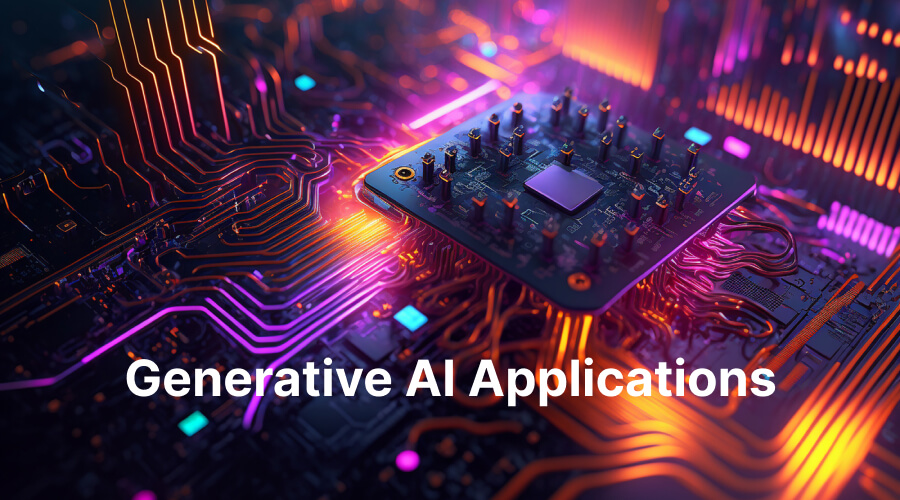
● Content Generation:
○ Text Generation: Generative AI models like GPT-3 can produce human-like text for various purposes, including article writing, creative storytelling, and chatbot interactions.
○ Image Generation: Generative models like DALL-E can create unique and imaginative images from textual descriptions, inspiring artists and designers.
○ Video Synthesis: AI-driven systems, such as deepfake technology, can generate synthetic videos, which has entertainment and potential misuse implications.
● Drug Discovery and Molecular Design:
○ Generative AI is used in drug discovery by predicting molecular structures with high potential for pharmaceutical applications.
○ AI models assist in identifying drug candidates and optimizing their properties, accelerating the development of new medicines.
● Autonomous Vehicles and Robotics:
○ Generative AI is pivotal in training autonomous vehicles and robots for various tasks.
○ It helps simulate real-world scenarios, generate synthetic data for training, and improve decision-making in self-driving cars and robotic systems.
● Natural Language Processing (NLP):
○ Generative AI enhances NLP applications with text generation and understanding capabilities.
○ Chatbots and virtual assistants like Google’s Duplex use Generative AI to engage in human-like conversations and perform tasks like making restaurant reservations.
These applications demonstrate the versatility of Generative AI across diverse domains, from content creation to complex scientific research, and its transformative impact on technology and innovation.
Generative AI Vs. Traditional AI: Challenges and Ethical Considerations
1. Challenges in Traditional AI
● Limited Adaptability:
○ Traditional AI systems struggle to adapt to new or unexpected situations. They are rigid and incapable of understanding from experience.
○ Any changes or updates to the system require manual adjustments to its rules.
● Dependency on Structured Data:
○ Traditional AI relies heavily on structured, well-organized data. It needs to work on unstructured or disorganized data, which is expected in the real world.
○ Handling unstructured data, such as natural language text or unformatted images, is a significant challenge.
● Scalability Issues:
○ As tasks become more complex, the rule-based approach becomes less effective. Managing a vast number of rules for intricate problems becomes impractical.
● Lack of Creativity:
○ Traditional AI lacks the ability to generate really innovative answers. It operates within the confines of predefined commands and practices.
○ Innovative problem-solving and creative content generation are beyond its capabilities.
● Inability to Handle Uncertainty:
○ Traditional AI struggles with uncertainty and ambiguity. It often fails when faced with incomplete or contradictory information.
○ Expert systems may provide inaccurate recommendations if the underlying knowledge base needs to be completed or updated.
● Data Quality and Bias:
○ Data quality is paramount in Traditional AI. Only precise data can guide good decisions or predictions.
○ Traditional AI may inadvertently perpetuate biases present in historical data, resulting in unfair or discriminatory outcomes.
● Maintenance and Updates:
○ Traditional AI systems require regular maintenance and updates to remain effective. As data and environments change, manual adjustments are needed.
○ This ongoing process can be resource-intensive and time-consuming.
Traditional AI, characterized by rule-based and expert systems, faces limitations in adaptability, scalability, creativity, and handling unstructured data. Data quality and bias challenges necessitate careful data management and ethical considerations.
2. Ethical Concerns with Generative AI
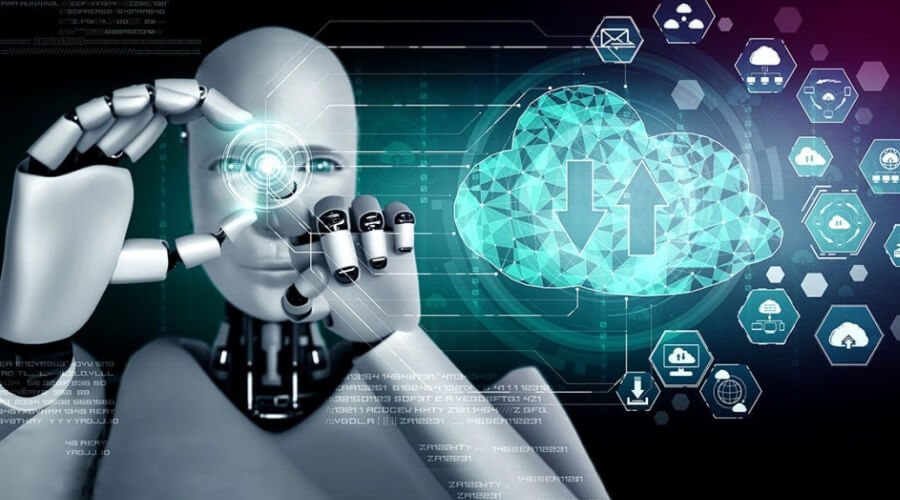
● Deepfake Generation:
○ Generative AI, particularly GANs, can create highly convincing deepfake videos and images that manipulate people’s appearances and actions.
○ This technology raises concerns about identity theft, privacy invasion, and the potential to create misleading or harmful content.
● Misinformation and Disinformation:
○ Generative AI can generate fake news articles, social media posts, and other content that can deceive the public.
○ Such misinformation can spread rapidly, eroding trust in information sources and causing real-world harm.
● Manipulation of Public Opinion:
○ Generative AI can manipulate public opinion by generating content that supports a particular narrative or agenda.
○ This raises concerns about the influence of AI-generated content on democratic processes and public discourse.
● Privacy Violations:
○ Generative AI can infringe on individuals’ privacy by creating synthetic content that compromises personal information or uses likenesses without consent.
○ This poses ethical dilemmas regarding consent and data protection.
● Bias Amplification:
○ If Generative AI models are trained on biased data, they may produce biased or unfair content, amplifying existing societal biases.
○ Addressing bias in AI training data and models is essential to mitigate these moral problems.
● Intellectual Property Issues:
○ Generative AI blurs intellectual property lines, as AI-generated content may replicate or mimic copyrighted material.
○ This raises queries about ownership, copyright infringement, and the rights of AI-generated content creators.
● Accountability and Attribution:
○ Determining responsibility for AI-generated content can be challenging, as it involves a combination of human inputs (training data, algorithms) and the AI’s actions.
○ Establishing clear accountability and attribution frameworks is essential to address ethical concerns.
Generative AI introduces ethical challenges related to deepfake generation, misinformation, privacy, bias, intellectual property, and accountability. Addressing these concerns requires a combination of technological safeguards, regulations, and moral policies to deliver trustworthy AI use.
Future Trends and Implications
1. The Evolving Landscape
● AI Development Trajectory:
○ AI is anticipated to persist in its quick growth, with increasing integration into various industries and daily life.
○ The trajectory involves more specialized AI models tailored for specific tasks and domains, driving greater efficiency and accuracy.
● Generative AI’s Role in Shaping the Future:
○ Generative AI will play a pivotal role in content creation, revolutionizing art, media, and marketing by generating high-quality, personalized content.
○ It will contribute to scientific discoveries, such as drug design, by accelerating research and modeling complex molecular structures.
● AI in Healthcare:
○ AI will continue to advance in healthcare, assisting in early illness detection, personalized medicine procedures, and drug development.
○ Telemedicine and AI-powered diagnostic tools will become more accessible.
● AI Ethics and Regulation:
○ Ethical concerns related to AI, particularly Generative AI, will lead to stricter regulations and guidelines for responsible AI use.
○ Clarity and responsibility in AI systems will be prioritized.
● AI and Robotics:
○ AI-powered robots will become more commonplace in manufacturing, healthcare, and agriculture, improving efficiency and reducing manual labor.
● Natural Language Processing (NLP):
○ NLP (Natural Language Processing) will see advancements in multilingual understanding, making AI more accessible globally.
○ AI-driven chatbots and virtual assistants will evolve to be more refined in understanding context and delivering meaningful interactions.
● AI in Education:
○ AI will transform education through personalized learning experiences, adaptive tutoring, and automated grading systems.
○ AI-powered tools will assist teachers in curriculum development and student support.
● AI in Sustainability:
○ AI will contribute to sustainability by optimizing resource allocation, energy management, and climate modeling.
○ Smart cities and AI-driven environmental monitoring will help mitigate the impacts of climate change.
● Quantum Computing and AI:
○ The intersection of quantum computing and AI will lead to breakthroughs in cracking complex problems that were previously intractable.
● AI in Space Exploration:
○ AI will enhance space exploration by autonomously controlling spacecraft, analyzing vast datasets, and assisting in extraterrestrial research.
The future of AI is promising and dynamic. Generative AI will shape content creation, while AI’s impact will be felt across diverse sectors, from healthcare to education and sustainability. Ethical considerations and regulations will guide AI development, providing trustworthy and valuable social integration.
2. Implications for Society
● Job Displacement:
○ Generative AI’s automation capabilities may lead to job displacement in specific industries, particularly those involving repetitive, rule-based tasks.
○ It necessitates reskilling and upskilling programs to transition the workforce to new roles.
● Creative Industries Transformation:
○ Generative AI’s influence on creative industries could redefine the role of human creators, raising questions about the authenticity and ownership of AI-generated content.
● Ethical Concerns:
○ The possibility for misusage of Generative AI, such as forming deepfakes and misinformation, underscores the demand for moral policies and responsible usage.
● Privacy Implications:
○ Generating synthetic content can infringe on individuals’ privacy, as AI can create highly realistic likenesses without consent, raising concerns about digital identity.
● Bias and Fairness:
○ Generative AI models may inherit biases present in training data, amplifying societal inequalities and discrimination in generated content.
● Regulations and Safeguards:
○ Policymakers must establish regulations and safeguards to ensure responsible AI use, encompassing data privacy, intellectual property, and content authenticity.
● Transparency and Accountability:
○ AI developers and organizations should prioritize translucence in AI systems, helping users comprehend when interacting with AI-generated content.
○ Clear accountability frameworks should be established to identify responsible parties in case of misuse.
● AI Education:
○ Society must invest in AI education and awareness to empower individuals to discern between AI-generated and human-created content and understand the ethical implications.
● International Cooperation:
○ Given the global nature of AI, international collaboration and standards will be essential to harmonize regulations and address emerging challenges.
○ Generative AI’s societal impact extends to job markets, creativity, ethics, privacy, and fairness. Regulations, safeguards, education, and responsible AI development will be critical to harness its potential while mitigating potential risks.
Conclusion
In the ongoing saga of Generative AI vs. Traditional AI, we witness the dawn of a new era where machines have mastered the art of analysis and become creators in their own right. Traditional AI, with its structured data and rule-based systems, paved the way for countless practical applications. Yet, its limitations in handling complexity and fostering creativity were evident.
Enter Generative AI, the creative powerhouse capable of producing art, music, and text, blurring the line between human and machine creation. Generative AI isn’t just a tool; it’s a catalyst for innovation, driving progress in content creation, healthcare, autonomous vehicles, and natural language processing. As these two AI paradigms coexist, it’s clear that Generative AI has reshaped the landscape, ushering in an era where the creative power of machines knows no bounds.