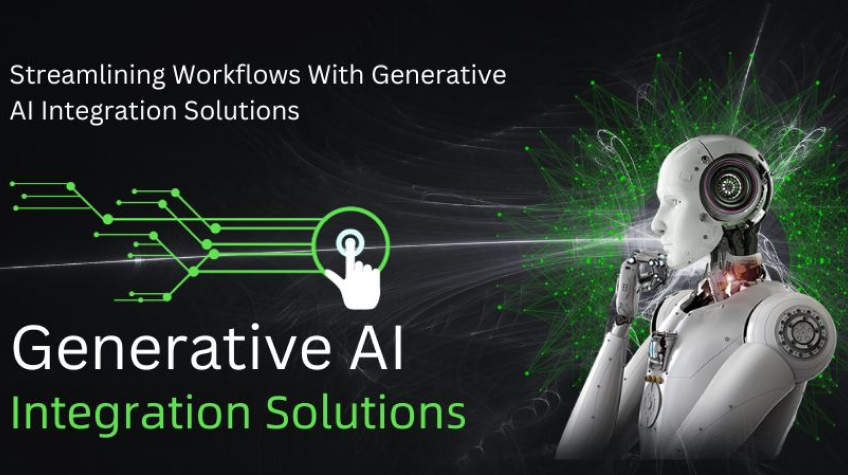
Generative AI Integration is ushering in a new era of autonomous content creation, insight discovery, and intelligent task automation. Models like DALL-E, GPT-3, and AlphaCode display unprecedented creativity in domains ranging from images to text, code, and more. However, thoughtfully integrating these emerging generative capabilities into real-world workflows remains a challenge. Specialized integration solutions can make generative AI accessible to enterprises in impactful yet controlled ways.
In this comprehensive guide, we will explore strategies for streamlining workflows through targeted deployment of generative AI integration solutions.
Generative AI’s Unique Value
Unlike traditional AI, which focuses on categorizing existing data, generative models create novel, realistic artifacts like text, code, media, and more. For example, GPT-3 crafts human-like text from simple prompts, while DALL-E 2 conjures photorealistic images from imagination. Their ability to learn patterns from vast datasets and synthesize new high-quality outputs autonomously constitutes a paradigm shift. Thoughtful integration of these generative capabilities can transform how organizations operate.
Also See : OpenAI launches Dall-E 3: Its Latest text-to-image Tool
Challenges With Adoption
While promising, effectively leveraging generative AI requires overcoming adoption hurdles. Models have specific interfaces and protocols requiring integration engineering for real-time invocation. Outputs may be unusable without filtering inappropriate content. Training datasets, model versions, and outputs require diligent tracking and governance.
Cost and computational resource management must be optimized, especially with large volumes. There are security considerations around access controls, data management, and monitoring. UX design is important for transparently blending AI capabilities into workflows. Specialized Data Integration Tools designed expressly for commercial usage can solve these challenges and streamline adoption.
Persona-Based Workflow Integration
Generative AI integration solutions tailored to different users’ needs enable workflow integration. For content teams, AI assistants autonomously produce wide varieties of high-quality text, visuals, and multimedia content dynamically customized for target personas and contexts. It amplifies content reach and relevance. Developers benefit from code generation suites integrating with IDEs to suggest context-aware boilerplate code and documentation. This accelerates software development. Data scientists tap intelligent data synthesis tools to autogenerate realistic datasets for more robust model training. This mitigates issues with small samples. Analysts leverage AI nowcasting dashboards that continuously generate projections based on the latest data. This enables real-time forecasting. With persona-based solutions, generative AI integrates seamlessly into various workflows rather than being siloed.
Formulating The Right Requests
Unlike conversational systems, effective prompting is key for generative AI. Some tips include providing relevant context and clear goals for generation upfront to guide the model. The scope should be bound by specifying the expected format, length, medium, etc. where possible. It is best to start with simple requests and build on previous outputs to refine results iteratively. If generation deviates from needs, examples of desired ideal outputs should be provided. For content, target persona, tone, call-to-action, and other authoring elements should be indicated. Well-formulated prompts are crucial for usable, aligned outputs from generative models.
Governance Guardrails
Like any powerful technology, prudent governance is required to harness generative AI responsibly. Human review processes screen computer-Automatically generated artifacts before usage based on risk levels. Watermarking and disclosure enable transparency where AI content is consumed publicly. Access controls, multi-factor authentication, and consent-based data flow prevent misuse. Ongoing model training audits detect skewed behavior or the emergence of bias. Mechanisms allow users to report inappropriate or abusive AI content. Periodic impact assessments weigh generative usage against ethical policies. With the right guardrails, generative models remain aligned with organizational values.
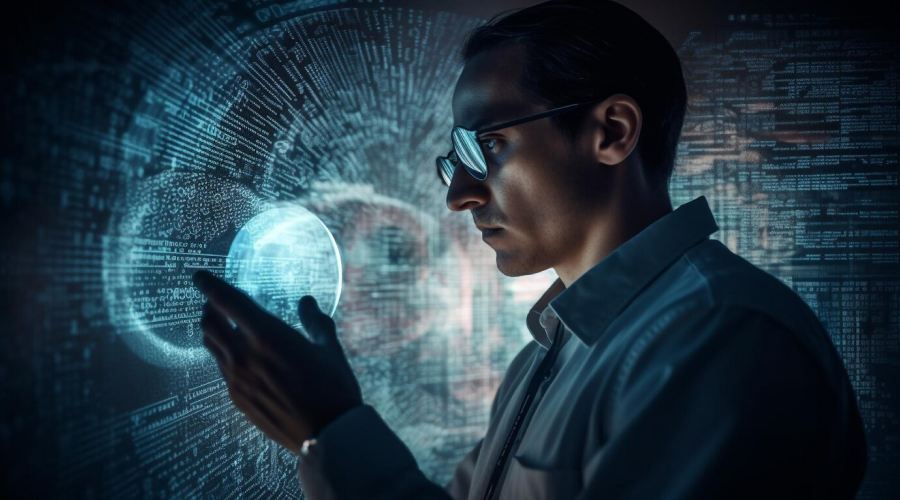
Evaluating Output Quality
Assessing output suitability both computationally and editorially ensures quality. Constraints can be set on technical quality – spelling, grammar, syntax, formatting, and data validity. Programmatic filtering leverages rules, ML classifiers, and natural language models. Subject matter experts review the accuracy, lack of bias, and appropriate content. Comparison against gold standard reference content is valuable for given domains. A/B testing the personalization of outputs to different personas enables refinement. User feedback loops provide insights into the utility and relevance of generated artifacts. Graduated real-world deployment from low to high-impact use cases will manage risk. Hybrid human+computer vetting maximizes generative solution value.
Accelerating Content Velocity
Generating content like social posts, comments, product listings, release notes, and more at enterprise scale saves significant time. Templates codify structures, while AI populates personalized content. Data-driven dynamic content remains evergreen with automatic updates. Mass personalization at scale creates tailored messaging for millions. Recommendation engines suggest high-probability content for scenarios. Notification and alert content triggered by data events stay relevant. Drafts can be completed for predictable content categories like earnings reports. With AI-powered content assembly, organizations publish at the speed of data.
Uncovering Insights
Generative AI helps analysts uncover non-obvious insights. It can reveal hidden correlations and causal relationships in data. Disparate data sources can be aggregated into summary briefings highlighting key developments. Interactive data stories and visualizations tailored to business goals can be delivered. Continuous production of projections across horizons leverages predictive models. Personalized data quality and integrity warnings refine analytics. Complex database queries and ETL transformations can be autocompleted. AI becomes the multiplier that takes analytics capabilities 10x rather than incremental improvements.
Automating Workflow Steps
Mundane yet crucial workflow steps are ideal targets for automation using generative AI. Gaps and issues requiring data resolution can be identified to improve analytics. Customer profiles can be matched with optimal products based on transaction history. Transactions requiring auditing can be flagged based on risk characteristics. Forms and requests can be routed to appropriate teams based on predicted categorization. Key events like customer support calls and meetings can be transcribed into log records. Documents like contracts can be compared and substantive differences summarized. Related content references can be suggested for web pages. Administrative data entry forms can be autocompleted. Precision workflow automation boosts employee productivity multifold.
Conclusion
Generative AI opens new possibilities for driving business value by enhancing human capacities. Targeted, purpose-built integration solutions can unlock these emerging capabilities for organizations while addressing adoption barriers. With thoughtful implementation following best practices around governance, quality control, and workflow integration, generative AI integration solutions will lead to higher levels of creativity, productivity, and growth across industries.