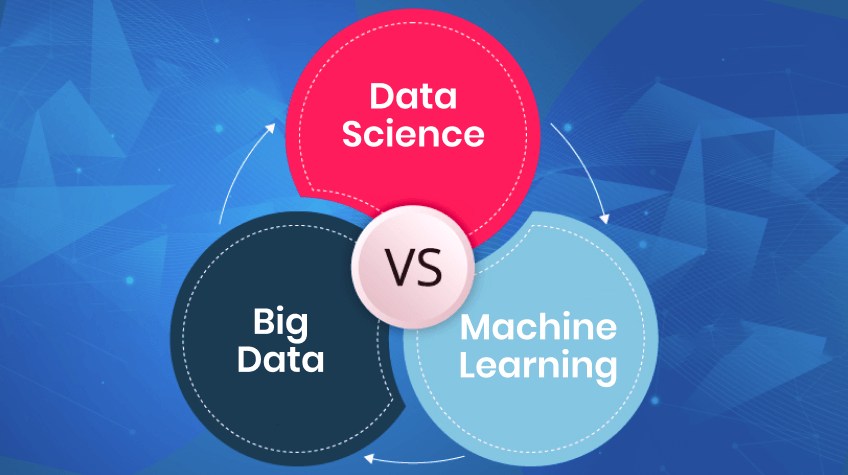
Data Science, Big Data, and Machine Learning are the hottest topics these days. Experts are talking a lot about these topics, and why not? After all, these three technologies have taken the world of business to the newest levels. Digging deeper into trending topics helps enhance your knowledge and elevate your standards. Today, you’re going to explore the most interesting comparison, which is data science vs big data vs machine learning.
Yes, these three are emerging fields in this technological realm. These fields are growing fields, and more and more professionals and graduates are choosing to work in these fields. If you’re a graduate or a professional who wants to switch careers, then utilize this guide and get paid highly.
This article gives you a detailed comparison of three trending fields, along with job opportunities, skills, and real-time examples. So don’t miss it! Without further delay, let’s dive in.
Data Science
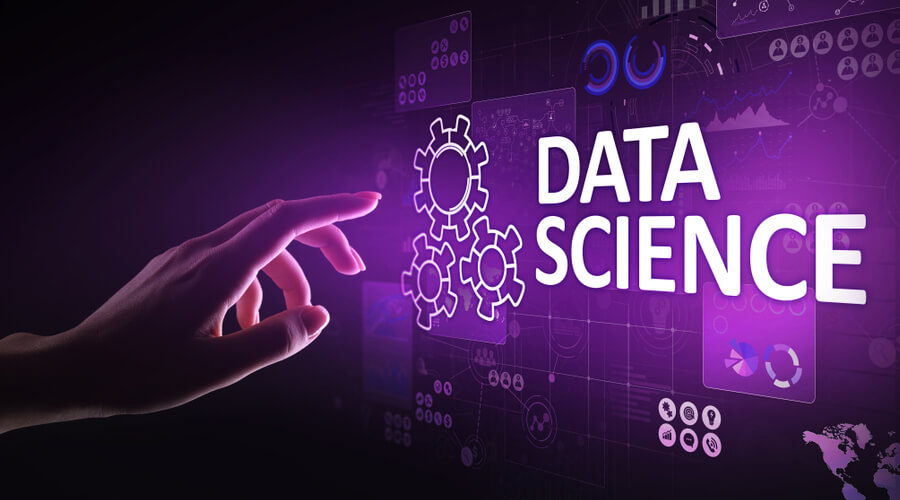
Data science is a multidisciplinary field that employs a range of methods and techniques to derive valuable insights and knowledge from data. It is one of the most sought-after fields that effectively addresses challenges and facilitates decision-making across a wide range of domains, including business, science, technology, medicine, and many others. It combines the principles and practices of computer engineering, artificial intelligence, statistics, and mathematics to analyze large amounts of data.
Data analysis is crucial to business success and creating diverse strategies. Plus, you can create predictive models, find patterns, and develop insights that leverage business on a successful path.
Data science necessitates a blend of skills and knowledge from diverse disciplines, including mathematics, statistics, computer science, programming, domain expertise, and effective communication. Also, it utilizes a wide range of tools and technologies, including Python, R, SQL, Excel, Tableau, TensorFlow, and more. This field is evolving at a rapid speed, presenting numerous opportunities for both data scientists and organizations.
Big Data
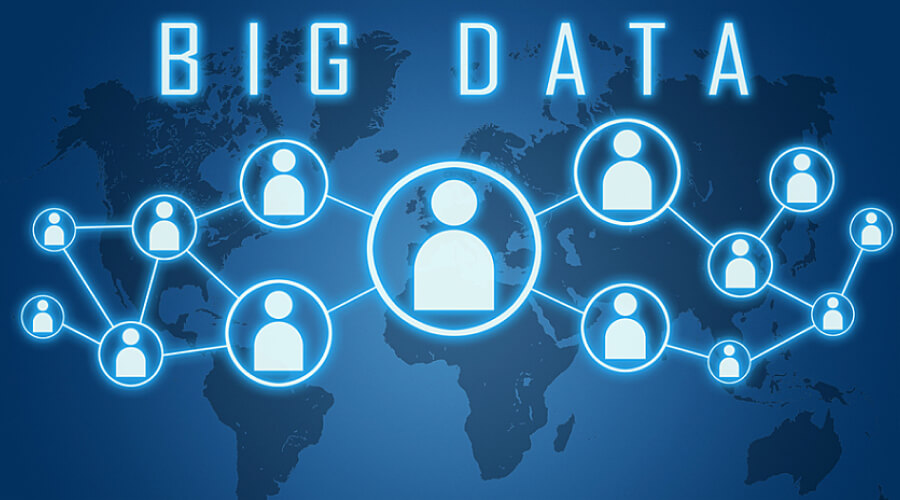
Big Data, as its name indicates, is a large and complex data set that is gathered from new data sources. The large data collection includes structured, unstructured, and semi-structured data. Big data can address challenges and facilitate decision-making across a wide range of fields, including business, science, technology, medicine, and others.
It is defined by three primary characteristics: volume, velocity, and variety. Volume refers to the quantity or amount of data, velocity refers to the speed at which data is generated and processed, and variety refers to the different types and formats of data.
This technology is used to analyze larger datasets to uncover valuable insights and patterns, leading to enhanced outcomes and performance. Using Big Data, businesses can greatly improve customer experience and satisfaction and optimize business processes and operations.
It is quite similar to data science, which analyzes the data to derive various strategies and lead a business on a successful path. Plus, it generates valuable insights to take the business to the next level.
Machine Learning
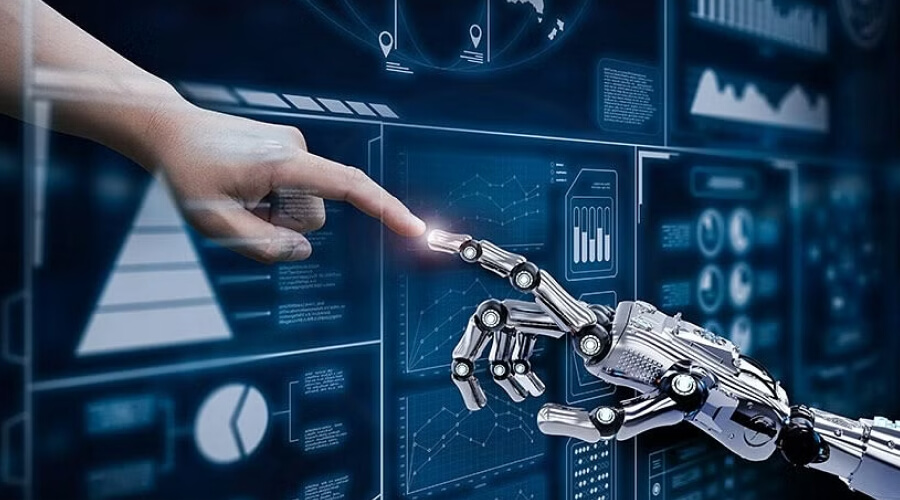
Machine learning is a branch of artificial intelligence. It utilizes algorithms and data to acquire knowledge from past experiences and carry out tasks that typically necessitate human intelligence. This technology is used in algorithms to extract data and then forecast future trends in a business or any industry. This subset of artificial intelligence allows machines to predict the future through the past activities of a user. The main objective of machine learning is to lead people on the right path.
Machine learning has a wide range of applications, including facial recognition, data analysis, outcome prediction, and content generation. Machine learning operates by following a series of steps, including gathering and organizing data from different sources, selecting and implementing an appropriate machine learning algorithm, training the algorithm using the available data in order to develop a machine learning model, evaluating and testing the model to determine its accuracy and performance when applied to new data, and finally deploying and updating the model.
Machine learning encompasses various types, including supervised learning, unsupervised learning, and reinforcement learning.
Data Science vs Big Data vs Machine Learning
From the above definitions, you can identify that each field has a distinct focus and is also interconnected. Now, let’s see how these three fields differ in this technological realm.
1. Applications
Discussing applications of data science, big data, and machine learning must be the top priority. Do you know why? It’s simple; you can understand it better. So, let’s start it.
➤ Data Science
Data science plays a crucial role in various fields, such as healthcare, transportation, sports, e-commerce, gaming, social media, finance, business, science and research, and search engines. In every field, experts leverage data science to analyze, optimize, automate, and improve the overall performance of these industries.
For instance, in healthcare, experts can analyze and predict disease using data science. It includes medical image analysis, tracking the menstrual cycle, personalizing treatment plans, identifying cancer tumours, etc. In parallel, data science is effectively used in other industries for better analysis and to enhance performance.
➤ Big Data
The importance of data is emerging in this digital world, and by using big data, experts can easily analyze and optimize complex data sets. Big data is used in several industries, including banking, communication, media, entertainment, healthcare, education, manufacturing, insurance, retail and wholesale trade, transportation, marketing, and the energy sector.
Here is an example to make this clear for you: Let’s take the media and entertainment industries. Big data is used in the media and entertainment industry to analyze customer data with behavioural data to create content according to a set of targeted audiences. Plus, you can analyze and gather insights from complex, large, structured (organized documents), semi-structured (like emails), and unstructured data (images or videos). Thus, big data is vital for analyzing and extracting insights from large data sets.
➤ Machine Learning
Machine learning is growing rapidly, day by day! Guess what? You’re using machine learning technology in your daily life. Yes, every individual embraces this technology unknowingly. Google Maps, Alexa, Google Assistant, etc. are some solid examples.
Various industries incorporate machine learning into their applications for performing specific actions. Till now, it has been used to recognize images and speech, predict traffic, recommend products, filter email spam and malware, drive self-driving cars, detect online fraud, perform stock market trading, diagnose medical conditions, and perform automatic language translation.
So, if you use machine learning technology in your devices or applications, you can easily perform such operations.
2. How does it work?
All three technologies perform many operations using data. From collecting data to deriving effective strategies, you can do various tasks with data science, big data, and machine learning. Let’s take a look at the working methodologies of these trending technologies.
➤ Data Science
Data Science uses various algorithms and techniques to gather data to extract insights and knowledge. Following these steps, data science provides actionable insights.
It includes data collection, data cleaning, exploratory data analysis, modeling, and interpretation. In data collection, experts gather raw data, remove errors, and organize the data in the cleaning phase. Then, they create predictive models using algorithms and, finally, analyze the results to derive actionable insights.
Data Science is very helpful for businesses and professionals. They use data science to derive successful strategies for business and other industries.
➤ Big Data
Big data analytics work in the following pattern: collecting, processing, cleaning, and analyzing large and complex data sets. Following this properly leads an organization or any sector on a successful path.
Collecting data refers to gathering structured, semi-structured, and unstructured data from various sources. The processing phase includes batch processing and stream processing to analyze the data and make quicker decisions. Cleaning data simply involves eliminating improper or irrelevant information from the gathered data.
Analyzing data includes data mining, predictive analytics, and deep learning. Ensuring these steps allows for easy analysis of complex data sets and provides valuable insights for business development.
➤ Machine Learning
Compared to data science and big data, machine learning is quite different. It is a subset of artificial intelligence. With this technology, you can develop algorithms, recognize patterns, make predictions, and take actions according to the data.
Types of machine learning are supervised learning, unsupervised learning, and reinforcement learning. Supervised learning is to make predictions or classifications; unsurprised learning is to extract patterns; and reinforcement learning is to make decisions.
Using these types of data, machine learning effectively analyzes and interprets vast amounts of data.
3. Advantages
You might be wondering what are the advantages of these three technologies. Well, in this section, you’re going to explore the advantages and importance of three fields. So, scroll down to know the advantages of all three technologies in the modern world.
➤ Data Science
Utilizing data science in business offers enormous benefits. It plays a crucial role in making decisions by extracting information and analyzing past data. Data science assists the organization in analyzing complex data, improving data quality, customizing and visualizing data, and using predictive analysis.
Other than this, data science offers extra insights that play an important role in reducing costs. It helps to make better business decisions, measures performance, deploys better products, and enhances the overall efficiency of the business.
Detailed Article: What is Data Science and its Benefits for Business
➤ Big Data
Big data helps to discover patterns, correlations, and trends. Utilizing appropriate big data tools to analyze and optimize complex and larger datasets can help you identify potential risks and enhance efficiency.
Businesses use big data to understand the behavioral patterns of customers and craft a custom-tailored strategy according to them. Plus, it is a cost-efficient way to elevate the overall performance of every industry. Therefore, harness the power of big data to witness improvements in business.
➤ Machine Learning
In general, machine learning offers various advantages. However, it provides enormous benefits in business applications; let’s know about them in detail. Using machine learning in an app offers data-driven insights, automation, detecting fraud and ensuring securities, giving a personalized customer experience, etc.
So, utilize this latest technology to enhance the overall security of the systems and address potential issues.
4. Skills Required
As everyone knows, skills are the most important thing to succeed in any career. Whether it is data science, big data, or machine learning, you must develop your skills accordingly to stand out from others.
➤ Data Scientist
Data is crucial. Businesses cannot perform well without proper data. This makes the role of a data scientist very crucial in an organization. A data scientist is one who leverages data to effectively address problems and challenges in a timely manner. Their job is not just limited to gathering and organizing data from different sources; they, in fact, need to select appropriate machine learning algorithms and statistical methods to effectively analyze data and uncover patterns, insights, and predictions to enhance the quality and performance of data or products.
To become a successful data scientist, you need to be well-versed in these technical skills. Make a checklist.Statistical Analysis and Computing
- Deep learning and machine learning
- Processing Large Data Sets
- Data Visualization and Data Wrangling
- Programming, Mathematics, and Statists
➤ Big Data Engineer
A big data engineer plays a crucial role in an organization. They develop, maintain, test, analyze, and evaluate a company’s data. Big data engineers collect a significant volume of data from various sources and establish a system that allows downstream users to access the data in a fast and efficient manner. In addition, they ensure the proper functioning and continuity of the data pipeline. They assist businesses by analyzing data to evaluate their performance, analyze market demographics, and forecast future changes and trends.
A big data engineer needs to possess a diverse range of skills and knowledge from multiple fields. These include mathematics, statistics, computer science, programming, domain knowledge, and communication.
Big Data Engineer needs to have the following technical skills to STAND OUT:
- Multi-Cloud Computing
- Data Visualization
- Machine Learning and Artificial Intelligence
- NoSQL
- DevOps, programming, and SQL
➤ Machine Learning Engineer
Machine learning engineer is one of the core job roles in this field. A machine learning engineer is a computer programmer who specializes in designing software that can autonomously run predictive models by leveraging extensive data sets. They collaborate closely with data scientists to analyze data and develop artificial intelligence (AI) systems that can enhance and improve a wide range of applications. These applications include chatbots, predictive text, driverless vehicles, and algorithms. They utilize programming languages such as R, Java, and Python to create software and enhance the efficiency of the learning process. Also, they indulge in research and development and assist businesses by introducing more advanced and user-friendly products and services.
Machine learning engineers must have a bachelor’s degree or higher in fields such as data science, statistics, computer science, or a related area.
- Below are some of the skill sets required to be a Machine Learning Engineer; take a look…
- Applied Mathematics
- Computer Science Fundamentals and Programming
- Machine Learning Algorithms
- Data Modeling and Evaluation
- Neural Networks and Natural Language Processing
5. Career Opportunities
Last but not least, checking career opportunities is a fundamental yet important thing when comparing unique fields. Choose a job role that enhances your creativity and knowledge. Plus, check the duties of the specified role and level up your professional life.
➤ Data Science
From the above-discussed points, you can conclude that data science is a promising domain with abundant opportunities. For sure, you can become a data scientist if you’re well-versed in data science. But other than this, which could be the best job role? After completing a data science course, you can choose any option from the list. Such as
- Data Analyst
- Data Architect
- Data Engineer
- Statistician
- Machine Learning Engineer
- Business Intelligence Analyst
- Data and Analytics Manager
- Database Administrator
You can consider choosing any option from this list and consult a professional in this technical realm.
➤ Big Data
Want to become a data scientist? But have you completed the big data course? No worries; you can become a data scientist even after pursuing a course in big data. Other than data scientists, you can choose a job role from this list. They are,
- Big Data Architect
- Big Data Engineer
- Business Analytics Specialist
- Data Visualization Developer
- Big Data DBA
- Big Data Administrator/Hadoop
However, every job role will be unique and have its own functionalities. Thus, prepare accordingly and move a step ahead in your professional life.
➤ Machine Learning
Undoubtedly, machine learning is the best option to seize great jobs in this advanced digital era. If you’re well-versed in machine learning, then you can become a data scientist or take on other roles. It includes,
- Machine Learning Engineer
- MLOps Engineer
- Data Science Consultant
- Machine Learning Research Scientist
- Computer Vision Engineer
- Machine Learning Instructor
- Business Intelligence Developer
- Research Engineer
- Algorithm Engineer
From this list, you can pick any role and shape yourself into an expert.
For sure, the above-mentioned jobs in every section have different responsibilities and duties. Hence, ensure that before finalizing the career option to avoid misconceptions after joining.
Data Science vs. Big Data
Both data science and big data are interconnected fields. If you want to become a data scientist, then you can excel in data science as well as big data.
Data science is an approach to extracting insights and knowledge from data, whereas big data refers to technologies and methods used to analyze larger, more complex data sets.
Big Data vs Machine Learning
Machine learning is quite different from big data because it is used to handle and manage large datasets from various types of data. On the other hand, machine learning is a technology that is utilized in developing algorithms to analyze data and make predictions according to the collected data.
However, these two fields are interconnected, so harnessing them elevates your business to the next level.
Data Science vs Machine Learning
Data science is a vast field, and machine learning is a part of this field. However, both have unique objectives. Machine learning allows machines to study data, recognize patterns, and make predictions to make custom-tailored decisions.
However, data science focuses on understanding, interpreting, and deriving insights from data to make data-driven decisions and tailored strategies.
Detailed Article: Data Science vs Machine Learning You Need to Know
Wrapping It Up…
So, there you have it: the comparison between the most trending fields: Data Science vs. Big Data vs. Machine Learning. Hopefully, this post has been informative for you. From this post, you can conclude that data science, machine learning, and big data are interconnected with other fields. Professionals in these fields frequently collaborate and develop distinctive, personalized strategies to help businesses excel. It is crucial to gain a comprehensive understanding of the methodologies and applications of data science, big data, and ML. Having comprehensive understanding of these three fields will help you excel in the digital realm. By doing so, you can position yourself as a top professional in this field.
Harness the power of these technologies to develop data-driven strategies tailored to specific industries. Moreover, acquiring knowledge of these advanced technologies presents you with vast career prospects.