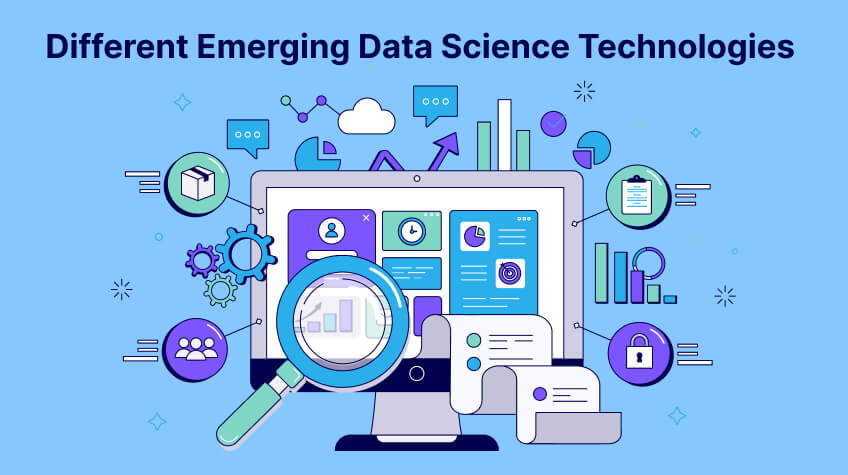
In recent years, data science has emerged as one of the most popular and quickly expanding sectors of technology. Data science technologies have improved significantly in response to the growing need for enhanced data analysis, predictive modeling, and artificial intelligence.
In this blog, we’ll look at some of the most recently developing data science technologies that are reshaping the profession.
But before that, you should have a look at our Data Science Course video for a better understanding.
1. Machine Learning that is Automatic (AutoML)
AutoML or Automated Machine Learning is a new data science tool that automates the process of developing machine learning models. This technique allows data scientists to focus on data analysis and interpretation rather than the time-consuming effort of model construction. AutoML solutions are meant to automate operations like feature engineering, hyperparameter tuning, model selection, and model deployment, decreasing the time and effort necessary to develop machine learning models.
2. AI that can be explained (XAI)
Explainable AI, or XAI, is a new developing technology that seeks to shed light on how AI models make decisions. It might be difficult to grasp how standard machine learning algorithms arrived at their judgments. XAI explains the underlying decision-making process, making it easier for humans to comprehend and trust AI model output. This technology is becoming increasingly significant as AI is used in key areas like healthcare and finance.
3. Databases Based on Graphs
Graph databases are a new form of database that is intended to store and analyze graph data structures. A graph database is a database that represents and stores data using nodes, edges, and attributes. This form of database is very beneficial in applications where data is heavily interrelated, such as social networks, recommendation systems, and fraud detection.
Here is another Data Science Training video that will help you understand the concepts in detail.
4. Edge Computing
Edge computing is a new technology that includes processing data at the network’s edge rather than in a central place. Edge computing allows for real-time data processing and decreases the delay associated with data transmission to a centralized site. This technology is becoming increasingly significant in applications requiring real-time decision-making, such as driverless cars.
5. Natural Language Processing (NLP)
Natural Language Processing, or NLP, is another developing data science technique that is altering our interactions with computers. Computers can read, interpret, and produce human language thanks to NLP. Chatbots, sentiment analysis, language translation, and speech recognition are all examples of how this technology is being used. In the age of big data, when analyzing and comprehending vast volumes of text data has become a need, NLP is becoming increasingly vital.
6. Federated Learning
Federated Learning is a new technique that allows machine learning models to be trained on dispersed data without requiring the data to be centralized in one location. This technique is especially beneficial when data is sensitive or cannot be transferred owing to privacy concerns. Data may be evaluated using Federated Learning without the need to share it with other parties, making it a powerful tool in fields such as healthcare and finance.
Related: What is Data Modeling? Its Types, Concepts and Techniques
7. Quantum Computing
Quantum computing is a new technology that employs quantum-mechanical processes to conduct data operations. This technique has the potential to revolutionize data science by allowing massive volumes of data to be processed in a fraction of the time that standard computers do. Although quantum computing is still in its early stages, it has the potential to transform areas such as banking, encryption, and drug development.
Related Blog: Explore Best Quantum Computers in the World
8. Blockchain Innovation
Blockchain technology is a new technology that is revolutionizing the way we store and distribute data. A blockchain is a decentralized database that is administered by a computer network. A blockchain stores data in blocks that are connected together in a chain. This technology is especially beneficial in applications requiring product traceability, such as supply chain management.
How is Data Science emerging?
Here are some of the ways in which data science is evolving:
Growing volumes of data: The rising amount of data accessible for analysis is one of the most significant advances in data science. Data is being created at an unprecedented rate due to the rise of the internet and the proliferation of sensors. As a result, new technologies and techniques for analyzing and processing data have emerged.
Machine learning advancements: Machine learning is a critical field of data science that has experienced substantial progress in recent years. Deep learning and other new algorithms are allowing robots to learn and improve their performance at an unprecedented rate. These developments are creating new possibilities for data scientists in industries such as healthcare, finance, and marketing.
The growth of cloud computing: The introduction of cloud computing has made vast volumes of data storage, processing, and analysis easier and more economical. Amazon Web Services, Google Cloud, and Microsoft Azure provide scalable and adaptable infrastructure for data science initiatives.
The advent of big data technologies: As data sets expand in size, new technologies emerge to assist in the management and processing of these massive data sets. Massive volumes of data can now be stored and processed effectively thanks to technologies like Hadoop, Spark, and NoSQL databases.
Data science integration into business: Data science is no longer viewed as a specialized discipline, but rather as an essential component of company operations. Several businesses are increasingly investing in data science teams and tools to better understand their consumers, products, and operations.
The emphasis on ethics and transparency: With the rising use of data science in sensitive domains such as healthcare and finance, there is a growing emphasis on ethics and openness in data science. As a result, there is an increasing need for data scientists who understand ethics and can guarantee that their work is open and responsible.
Conclusion
To summarize, the discipline of data science is fast growing, and new tools to enable data gathering, processing, analysis, and visualization are continually emerging. We have looked at some of the developing technologies that are impacting the future of data science, such as blockchain, edge computing, federated learning, graph databases, and natural language processing, in this blog.
As the area of data science evolves, it is critical for data professionals to keep current on emerging technologies and to continue to enhance their skills and knowledge. By adopting these new technologies, data scientists can unleash the full potential of data and drive innovation across a wide range of industries and applications.